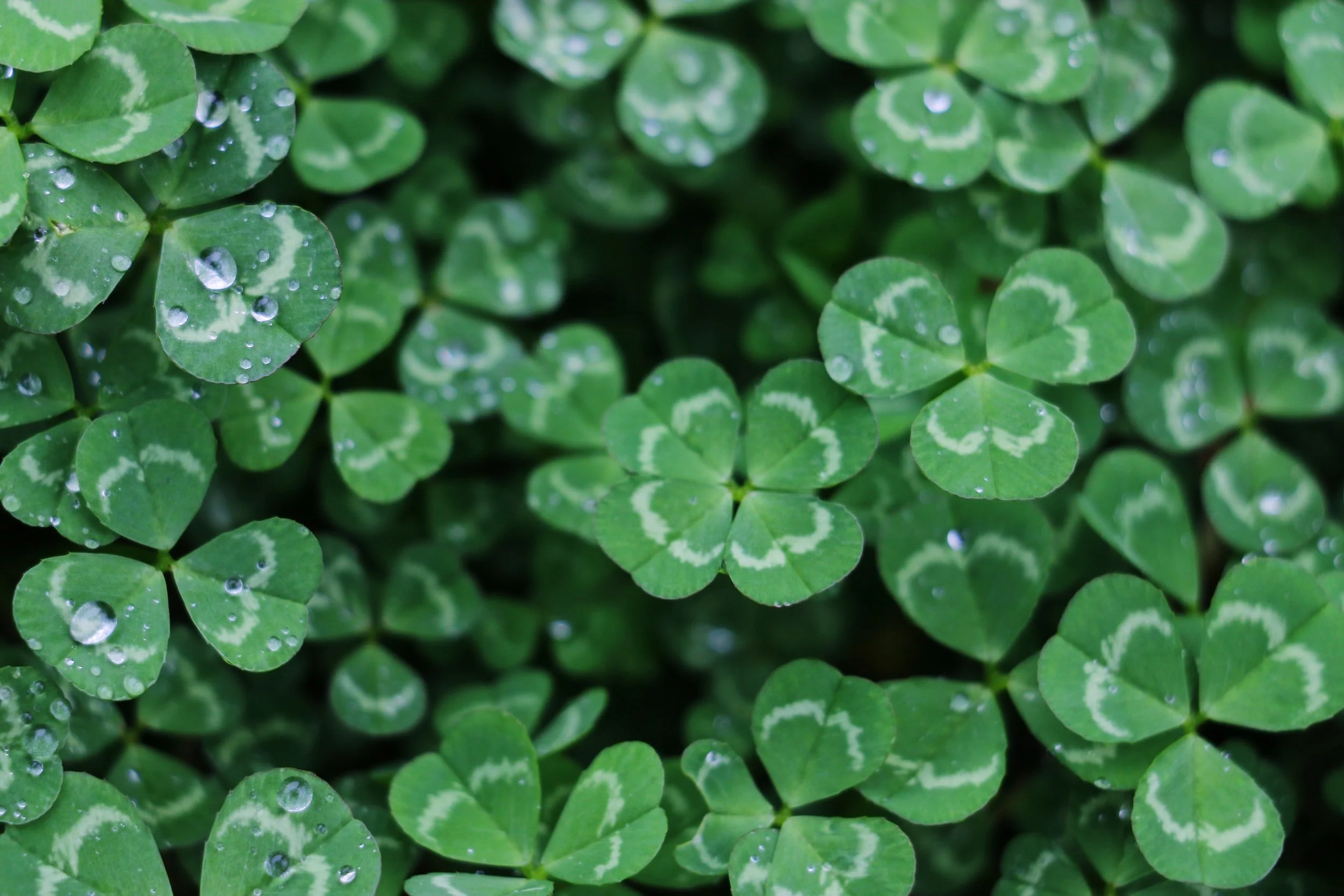
Research Areas
-
Bayesian methods grant researchers a high degree of effectiveness in modeling messy data. They have been shown to outperform traditional methods for small-sample problems. They are relatively less mathematically demanding for complex model estimations involving multilevel structures, missing data, and nonnormal data analysis. In some cases, Bayesian methods are the only viable option for new types of models and analysis.
Recent work:
*Kim, S., §Tong, X., Zhou, J. & Boichuk, J. P. (2021). Conditional Median based Bayesian Growth Mixture Modeling for Nonnormal Data. Behavior Research Methods. DOI: 10.3758/s13428-021-01655-w.
Tong, X. (2021). Semiparametric Bayesian Methods in Growth Curve Modeling for Nonnormal Data Analysis. Journal of Behavioral Data Science. DOI: 10.35566/jbds/v1n1/p4.
Ke, Z., & §Tong, X. (2021). Correcting for the Multiplicative and Additive Effects of Measurement Unreliability in Meta-Analysis of Correlation. Psychological Methods. DOI: 10.1037/met0000396.
Tong, X., & Ke, Z. (2021). Assessing the impact of precision parameter prior in Bayesian nonparametric growth curve modeling. Frontiers in Psychology. A special research topic on Moving Beyond Non-Informative Prior Distributions: Achieving the Full Potential of Bayesian Methods for Psychological Research. DOI: 10.3389/fpsyg.2021.624588.
-
Longitudinal data are widely collected in practice. With longitudinal data, one can study intraindividual changes over time and interindividual differences in intraindividual changes. Although normal-distribution-based maximum likelihood has been widely used for model estimation, its desirable properties break down with the involvement of nonnormal data. Without careful consideration of the nonnormality issue, model estimation results may be biased, and statistical inferences can be improper. Unfortunately, practical data in social and behavioral sciences are typically nonnormally distributed and pose great challenges to real data analysis.
An important line of our research is to develop methods to properly handle nonnormal longitudinal data, including data containing outliers, proportion data, skewed data, text data, etc
.
Recent work:
*Zhang, T., Tong, X., & Zhou, J. (2022). Disentangling the influence of data contamination in growth curve modeling: A median based Bayesian approach. Journal of Behavioral Data Science. DOI: 10.35566/jbds/v2n2/p1..
Tong, X., *Zhang, T., & Zhou, J. (2020). Robust Bayesian Growth Curve Modeling using Conditional Medians. British Journal of Mathematical and Statistical Psychology. DOI: 10.1111/BMSP.12216.
Tong, X., & Zhang, Z. (2020). Robust Bayesian Approaches in Growth Curve Modeling: Using Student's t Distributions versus a Semiparametric Method. Structural Equation Modeling. 27, 544-560. DOI: 10.1080/10705511.2019.1683014.
Tong, X., & Zhang, Z. (2017). Outlying Observation Diagnostics in Growth Curve Modeling. Multivariate Behavioral Research, 52, 768-788. DOI: 10.1080/00273171.2017.1374824.
-
Missing data in social and behavioral sciences is considered to be the norm, not the exception. We have contributed to the missing data analysis literature in the study design phase as well as the data analysis phase.
Recent work:
*Shi, D., & Tong, X. (2020). Mitigating Selection Bias: a Bayesian Approach to Two-stage Causal Modeling with Instrumental Variables for Nonnormal Missing Data. Sociological Methods & Research. DOI: 10.1177/0049124120914920.
*Mazen, J. A. M., & Tong, X. (2020). Bias Correction for Replacement Samples in Longitudinal Research. Multivariate Behavioral Research. DOI: 10.1080/00273171.2020.1794774.
Taylor, L. K., Tong, X., & Maxwell, S. E. (2020). Evaluating Supplemental Samples in Longitudinal Research: Replacement and Refreshment Approaches. Multivariate Behavioral Research. 55, 277-299. DOI: 10.1080/00273171.2019.1628694.
*Mazen, J. A. M., Tong, X., & Taylor, L. K. (2019). Evaluation of Supplemental Samples in Longitudinal Research with Nonnormal Missing Data. Behavior Research Methods. 51, 1321-1335. DOI: 10.3758/s13428-018-1070-3.
-
With the extraordinary support of a collegial environment at UVA, we have developed collaborations with a number of researchers on Grounds and across universities.
Our substantive research interests include longitudinal studies of cognitive ability and achievement skills, healthcare analytics, sustainability research, etc.
Recent work:
*Dela-Cruz, K. L., Kelsey, C. M., Tong, X., & Grossmann, T. (2023). Infant and Maternal Responses to Emotional Facial Expressions: A Longitudinal Study. Infant Behavior and Development. DOI: 10.1016/j.infbeh.2023.101818.
*Womack, S. R., Wilson, M. N., Tong, X., Lemery-Chalfant, K., & Shaw, D. S. (2022). Trajectories of Early Childhood Family Instability and the Development of Externalizing Behaviors from Middle Childhood to Adolescence: A Prospective Study of At-risk Families. Child Development. DOI: 10.1111/cdev.13726.
Snyder, A. L., Tong, X., & Lillard, A. S. (2021). Standardized Test Performance in Public Montessori Schools. Journal of School Choice: International Research and Reform. DOI: 10.1080/15582159.2021.1958058.
Ren, L., Tong, X., Xu, W., Wu, Z., Zhou, X. & Hu, B. Y. (2021). Distinct Patterns of Organized Activity Participation and Their Associations with School Readiness among Chinese Preschoolers. Journal of School Psychology. 86, 100-119. DOI: 10.1016/j.jsp.2021.03.007.